Leadership
Exploring the intersection of AI and a human-centric future
Published
6 months agoon
By
Dimpal Bajwa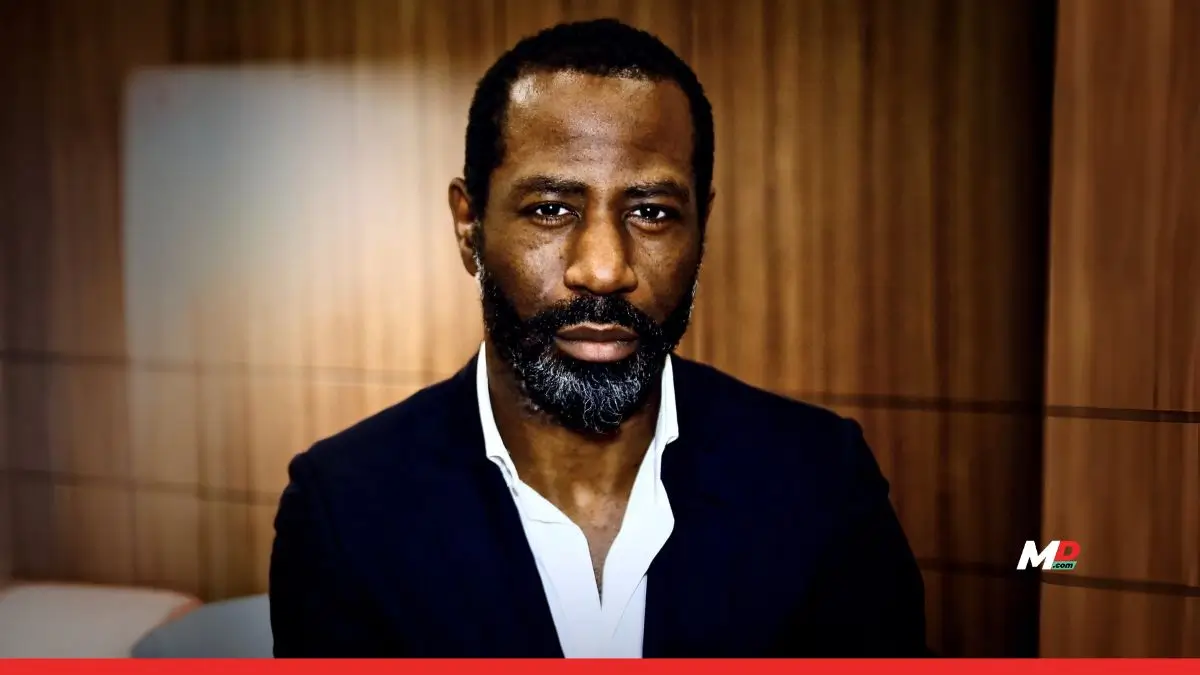
Imagine artificial intelligence that operates with unwavering integrity – systems that naturally adapt to different cultures, values, and situations while overcoming biases and keeping humans at the center. This is what Hamilton Mann calls “Artificial Integrity.”
Mann, who combines experience as a digital strategist, technologist, researcher, management expert, and business leader, argues that the real challenge with AI isn’t just making it smarter – it’s ensuring it consistently demonstrates integrity in its operations.
In his work, Mann explores how traditional ethical frameworks fall short when applied to modern AI systems. He presents new approaches for developing AI that people can genuinely trust as these technologies become increasingly influential in our lives.
Dive into the conversation, as we catch up with him. Grab a cuppa and settle in!
Your book emphasizes the need for AI systems to prioritize integrity over intelligence. Can you elaborate on how “artificial integrity” differs from traditional ethical AI frameworks and why this distinction is critical for the future of AI?
Artificial integrity represents a paradigm shift from traditional ethical AI frameworks by addressing a critical gap in how we think about AI systems. Most ethical AI frameworks focus on compliance—rules, guidelines, and reactive safeguards to prevent harm. While necessary, these frameworks often lack the proactive mechanisms required for AI to exhibit human-centered behavior consistently and autonomously.
Artificial integrity, on the other hand, is about embedding integrity-led reasoning into the very fabric of AI systems. It’s not just about preventing unethical outcomes but ensuring that the AI actively aligns its actions with human values, even as it evolves in unpredictable ways. Unlike traditional approaches, which often treat ethics as an external layer or afterthought, Artificial integrity integrates the principles of responsibility, fairness, and societal impact as core functionalities of the AI’s decision-making process.
This distinction is critical for the future of AI because as AI systems grow more autonomous and adaptive, their actions will increasingly influence complex societal dynamics.
We need systems that not only follow rules but also ‘choose’ to act in ways demonstrating something akin to what we called integrity to foster trust, inclusion, and equity—even in ambiguous scenarios. Artificial integrity systems can bridge the gap between technological advancement and human well-being, ensuring that AI serves as a partner in creating a sustainable and ethical future, rather than amplifiying existing flaws in our societies systems.
This is at the heart of what I call artificial integrity.
Social media platforms and any system widely spreading content at an unparalleled viral velocity must embrace artificial integrity as a new foundational paradigm of system design.
The need for artificial integrity—an AI’s ability to consistently recognize the ethical, moral, and social implications of the influence and outcomes of widespread online mass-generated content (e.g., social media platforms), including its own generation of content and outcomes (e.g., AI chatbots, AI agents)—learning both ex-ante (proactively) and ex-post (reflectively) from experience to guide decisions and actions that reflect integrity-driven behavior in a context-sensitive manner—is an increasingly critical imperative as the entrenchment of AI systems, including social media, in the very fabric of our society continues to evolve from life-neutral to life-altering, and in some unfortunately already occurring cases, life-ending.
One of the key promises of artificial integrity is its ability to adapt to diverse cultural contexts and value models. How can AI systems effectively achieve this adaptability?
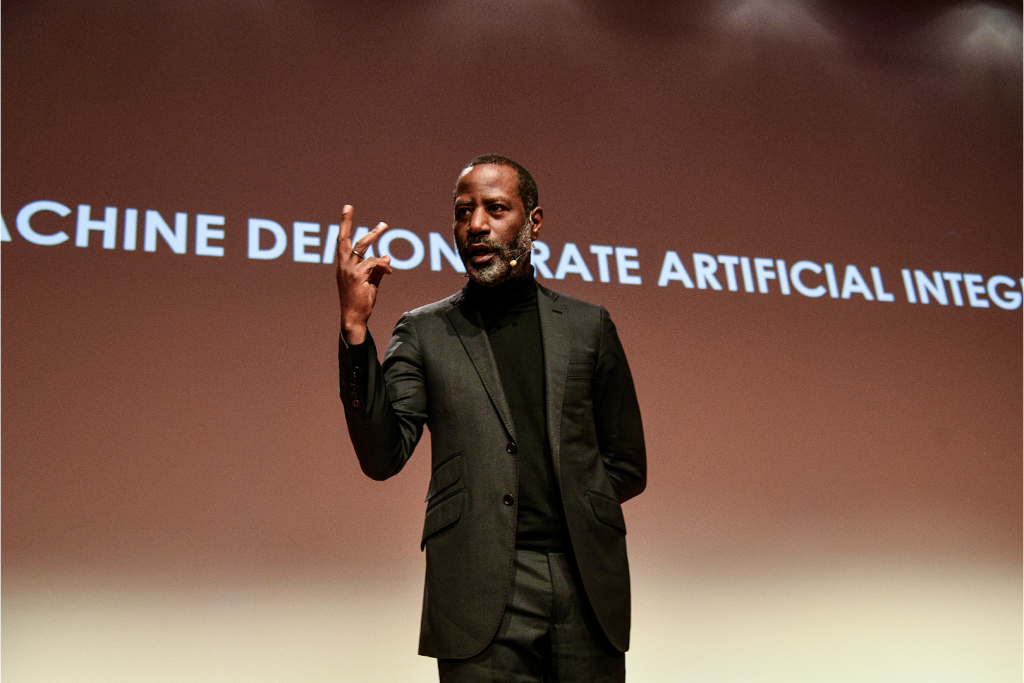
Adapting to diverse cultural contexts and value models is indeed one of the foundational promises of artificial integrity.
A few mechanisms can be considered toward achieving a certain level of this capability. For example, this adaptability can be achieved through a combination of several key elements embedded into the AI system’s design and operational framework.
First, AI systems must be trained on datasets that are representative of the cultural contexts they will serve. This involves incorporating local languages, customs, ethical norms, and value systems into the training data. By doing so, the AI can learn to recognize and respect culturally specific nuances, ensuring that its outputs are not only relevant but also aligned with local expectations.
Context-aware learning frameworks play also a pivotal role in ensuring AI systems can adapt to diverse cultural environments. These frameworks enable AI to dynamically assess the cultural, social, and historical context in which it operates and tailor its behavior accordingly. By continuously analyzing environmental cues, user interactions, and contextual feedback, the AI can refine its responses in real time to remain relevant and respectful.
A context-aware framework in AI is not just code or a specific training method, but rather a comprehensive approach to designing and implementing AI systems encompassing multiple aspects such as, architecture 58, data processing 4 , machine learning techniques 63, algorithms 9, integration 17, and even application design 2.
AI systems designed with such frameworks can dynamically adjust their behavior based on the context in which they operate. These frameworks incorporate sensors, contextual data, and scenario-based learning to tailor decisions. For example, a conversational AI might adjust its tone, formality, and phrasing depending on the cultural norms of the user’s location. A medical diagnostic AI could adapt its recommendations based on local healthcare protocols and available resources.
Another complementary approach is the use of modular integrity frameworks that can be adjusted to fit different cultural contexts. AI systems need to be equipped with mechanisms to prioritize integrity-driven considerations dynamically, based on the specific cultural or societal values they are engaging with.
A modular integrity framework is not just a set of predefined rules or guidelines but a flexible and adaptable system that integrates ethical principles into every stage of the AI lifecycle. These frameworks encompass ethical architecture design, decision-making algorithms, real-time rule adaptation, and dynamic prioritization of ethical principles.
Modular integrity frameworks are not just a set of predefined rules or guidelines but flexible and adaptable systems that aim to guide AI systems in delivering integrity-led outcomes or mimicking integrity-driven behavior within defined boundaries.
AI systems designed with modular integrity frameworks can dynamically adapt their ethical reasoning and decision-making processes based on the context in which they are deployed to a certain degree. For instance, an autonomous vehicle could prioritize pedestrian safety differently based on the local traffic laws and urban infrastructure. These frameworks enable AI to navigate complex ethical landscapes by embedding culturally and contextually specific decision-making capabilities, ensuring that the system’s actions align with the integrity-driven expectations of the communities it serves.
Adaptability to cultural norms and value models can also be achieved through continuous learning capabilities enabled by structured feedback loop mechanisms. By collecting feedback from end-users and monitoring the ethical implications of their actions, these systems can refine their behavior over time to better align with cultural expectations.
These mechanisms integrate real-time user feedback, automated monitoring systems, and adaptive algorithms to ensure that the AI remains aligned with its operational and integrity goals.
For example, a customer service chatbot could learn to adjust its conversational style based on cultural preferences, such as using more formal language in regions where respect for hierarchy is emphasized, or adopting a friendly, casual tone in areas where informality is preferred. Similarly, a recommendation system for digital content could refine its suggestions to avoid culturally sensitive topics or prioritize content that aligns with local traditions and values. These mechanisms ensure that AI systems remain respectful and relevant within diverse cultural contexts.
In essence, artificial integrity systems achieve adaptability to diverse cultural contexts and value models by combining technological sophistication with deep cultural awareness. This requires engaging diverse stakeholders—including ethicists, sociologists, and representatives from local communities—throughout the development and deployment phases. This collaborative approach ensures that the AI reflects a plurality of perspectives and values, rather than imposing a singular, potentially biased, worldview.
Having said that, more research and investment needs to be made for artificial integrity to advance further in maturity in designing AI systems capable of adapting to diverse cultural contexts and value models.
This is a critical condition for ensuring AI systems can operate responsibly, upholding integrity with regard to social expectations in the diverse environments where they are deployed.
While the above-described approaches and frameworks are currently mostly limited to operating within established rule sets and known contexts, future advancements could enable the development of artificial integrity with a greater ability to cope with higher degrees of uncertainty. For example, applying heuristics and probabilistic reasoning could help AI systems navigate situations where explicit rules are incomplete or undefined, ensuring that their actions remain aligned with overarching integrity goals.
As we increasingly delegate decisions to AI systems deeply entrenched in the core functioning of societal infrastructures, we are simultaneously making these vital systems more reliant on AI for their operation and service continuity. This growing dependence weaves an intricate entanglement between our daily lives and the expanding ecosystem of ‘AI-of-things.’ In this context, advancing AI systems to prioritize integrity-led outcomes as their foundational principle—viewing intelligence as an enabler toward that end, rather than raw performance for its own sake—becomes not just desirable but indispensable.
Only by anchoring these systems in integrity can we ensure that they uphold the stability and reliability of the critical frameworks that sustain modern society.
What are some of the biggest challenges businesses and policymakers face when designing and implementing AI systems aligned with the principles of artificial integrity? How can these hurdles be overcome?
One of the biggest challenges businesses and policymakers face when aligning AI systems with the principles of artificial integrity is overcoming the entrenched ‘optimization-first’ mindset that dominates AI design.
AI systems are traditionally built to maximize efficiency, profitability, or user engagement—metrics that often overshadow deeper considerations of ethical alignment, cultural adaptability and so integrity.
Shifting this paradigm requires rethinking the fundamental purpose of AI systems, moving from a mindset of ‘intelligence maximization’ to ‘integrity prioritization.’ This shift is not just a technical or policy challenge; it is both. It is a techno-social-cultural challenge that involves redefining success metrics for AI projects, not from an ‘itemic’ perspective, looking at one isolated item, whether it could be technological, environmental, economic, social, or political, but from a systemic standpoint.
Another significant challenge lies in the fragmented understanding of integrity across global cultures.
Many existing approaches attempt to establish universal principles, but these can unintentionally marginalize local nuances.
Artificial integrity calls for the development of dynamic, modular integrity-led systems that can respect and adapt to these nuances. Achieving this balance demands interdisciplinary collaboration, blending expertise from sociologists, anthropologists, ethicists, and technologists.
Finally, a less-discussed hurdle is the issue of trust asymmetry. Policymakers and businesses often assume that AI systems will be trusted if they are ‘explainable’ or ‘transparent,’ but trust is inherently contextual.
In many cases, communities may mistrust AI not because they don’t understand it, but because they perceive it as being imposed by external actors with misaligned interests.
To overcome this, businesses and policymakers need to engage deeply with local communities, not just as end-users but as co-creators of AI systems, fostering a sense of ownership and shared purpose.
Overcoming these challenges requires to recognize that AI’s ultimate promise lies not in automating tasks or predicting outcomes but in amplifying human values—values that are diverse, evolving, and context-dependent.
You argue for a future where AI enhances human capabilities rather than replaces them. What strategies do you suggest for ensuring that AI remains human-centered, particularly in sectors with high stakes like healthcare, aerospace or transportation?
Ensuring that AI remains human-centered, particularly in high-stakes sectors like healthcare, defense, or governance, requires a strategic alignment with the four modes of artificial integrity: Marginal, AI-First, Human-First, and Fusion. Each mode plays a critical role in designing and deploying AI systems that enhance human capabilities while upholding integrity.
- Marginal Mode is applicable in situations where neither human nor AI plays a dominant role, as the tasks involved are relatively low-stakes or procedural. For instance, AI can automate routine administrative functions like document sorting or scheduling, with humans ensuring basic compliance standards. In healthcare, this might include using AI to manage patient appointment scheduling or automate the organization of medical records—tasks that require neither clinical expertise nor ethical deliberation. This selective involvement preserves resources for more critical tasks while maintaining human oversight and avoiding unnecessary over-reliance on AI.
- AI-First Mode is applicable in situations where AI plays a dominant role due to its superior speed, precision, and ability to handle complex, data-intensive tasks that exceed human capabilities. For instance, AI can be deployed to optimize flight paths in real-time, factoring in variables like weather conditions, air traffic, and fuel efficiency, with minimal human intervention. In aerospace, this might include AI-powered autonomous systems for spacecraft navigation, enabling precise trajectory adjustments during missions where human reaction times or decision-making would be insufficient. This strategic reliance on AI enhances performance and operational efficiency while ensuring safety through carefully designed oversight mechanisms.
- Human-First Mode is applicable in situations where human intelligence and judgment play a dominant role, with AI serving primarily as a supportive capability to augment decision-making and improve efficiency. For instance, AI can assist drivers or transportation planners by providing real-time traffic updates, route optimization, or predictive maintenance alerts, while humans retain ultimate control and responsibility. In transportation, this might include advanced driver-assistance systems (ADAS) that provide lane-keeping support or collision warnings, empowering drivers to make better decisions without replacing their expertise. This approach ensures that human agency and accountability remain central, with AI acting as a reliable enabler to enhance safety and convenience.
- Fusion Mode is applicable in situations where human and artificial intelligence collaborate seamlessly, leveraging their respective strengths to achieve outcomes that neither could accomplish alone. For instance, Brain-Computer Interfaces (BCIs) in healthcare enable individuals who have lost a limb to regain functionality by combining AI’s processing power with human neural input. AI interprets electrical signals from the brain, translating them into precise movements for a robotic prosthetic arm or leg. Meanwhile, the human user provides continuous feedback, adapting and refining the system’s responses to ensure natural and intuitive control. This collaboration empowers patients to regain independence and mobility, showcasing the transformative potential of Fusion Mode in healthcare.
The next frontier is AI systems capable of switching from one mode to another depending on context, allowing situational adaptability required for AI systems to demonstrate a greater level of artificial integrity.
As a global leader in digital transformation, what role do you think leaders in technology and business need to play in advancing the concept of artificial integrity and fostering a trust-driven AI ecosystem?
The rule in business has always been performance, but performance achieved at the cost of amoral behavior is neither profitable nor sustainable.
Warren Buffett famously said, ‘In looking for people to hire, look for three qualities: integrity, intelligence, and energy. But if they don’t have the first, the other two will kill you.’
The same principle applies to the AI systems we bring into our businesses.
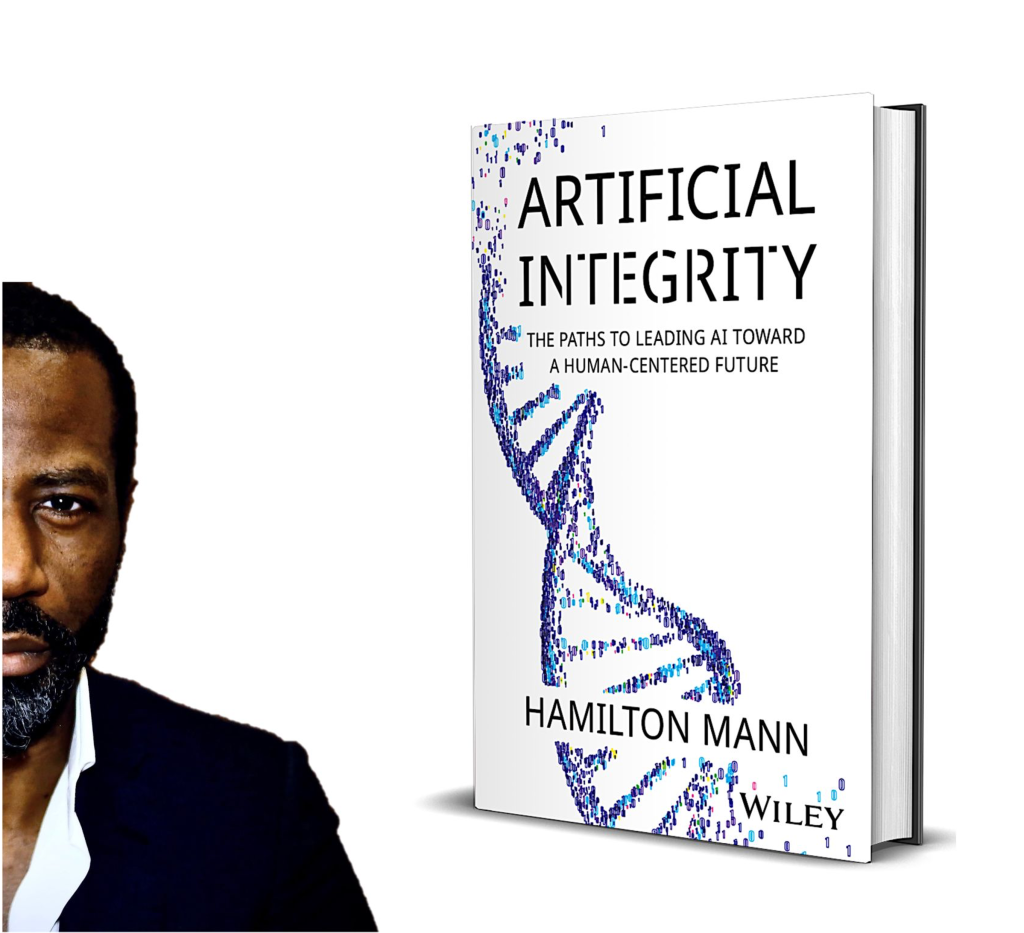
Leaders must ensure that the AI systems they adopt are not only mimicking intelligence but also demonstrating a form of integrity—an ability to make decisions that align with ethical, moral, and social values.
Leaders who embrace artificial integrity position themselves as stewards of responsibility, accountability, and foresight. By doing so, they avoid the pitfalls of ‘ethical washing’—a superficial commitment to ethics that can erode trust and ultimately threaten their organization’s very existence.
The urgency for artificial integrity oversight to guide AI in businesses is not artificial—it is real and profoundly beneficial for both business and society.
Artificial integrity is the new AI frontier.
You may like
-
CXO Movements: Tech Giants Make Strategic Leadership Changes
-
Six Titans Just Placed a $30 Billion Bet on AI’s Future
-
What Does China’s Recall of Foxconn Engineers Mean for Indian Manufacturing and Consumers?
-
War Hero to Healthcare Visionary, Lt. Gen. Ashok Kumar Jindal Inspires IIM Raipur’s New Batch of Emerging Managers Bootcamp
-
PM Modi’s Historic Ghana Visit Deepens Strategic Partnership and Expands India-Africa Cooperation
-
India, US on Brink of Interim Trade Deal as July 9 Tariff Deadline Nears